Explainable AI
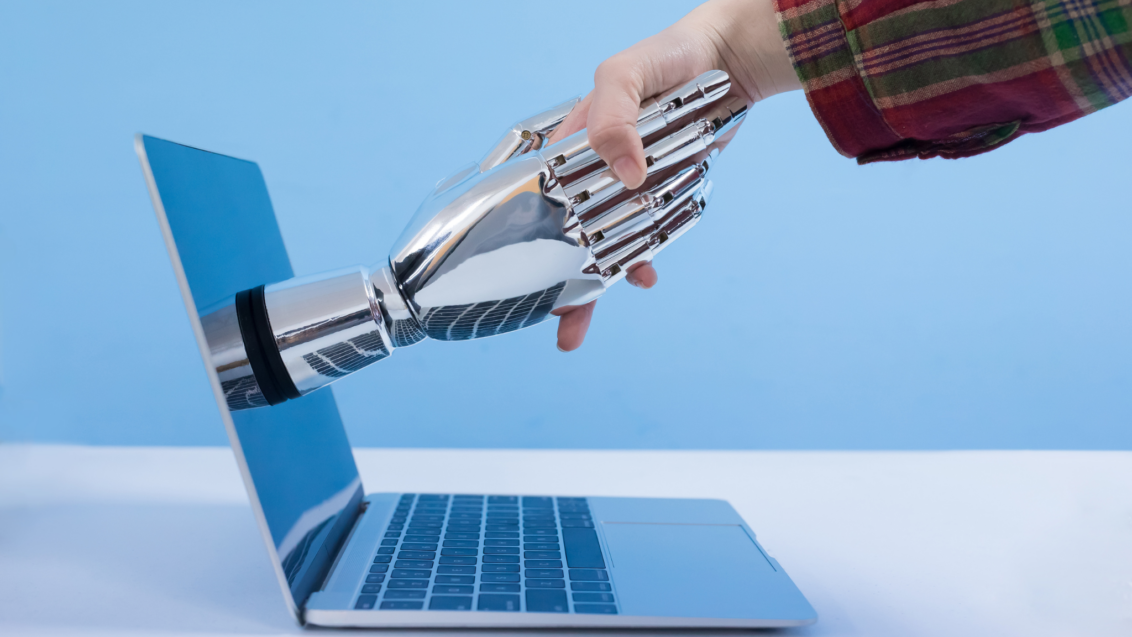
Understanding the importance of transparency in Artificial Intelligence
In recent years, Artificial Intelligence (AI) has advanced considerably, touching various domains like autonomous vehicles and personalized healthcare. Yet, the growing integration of AI into our routines raises apprehensions regarding the opacity of its decision-making processes. Explainable AI, a burgeoning research field also known as XAI, strives to create AI systems with greater transparency in decision-making and seek to elucidate the workings of non-transparent models.
What is Explainable AI and why is it important?
Explainable AI, a subset of artificial intelligence, focuses on explaining algorithms and techniques to elucidate the decision-making mechanisms of AI systems. Essentially, Explainable AI aims to bridge the gap between the non-transparent nature of AI algorithms and the imperative for transparency and accountability in decision-making processes.
Meanwhile, Natural Language Processing (NLP) is reshaping the technological landscape, enabling automation of tasks and extraction of insights from extensive datasets. NLP innovations, spanning from text classification to voice assistants, have transformed human-machine interactions, empowering machines to comprehend human language and provide intelligent responses.
The necessity for explainable AI arises from the quest for transparency and accountability in AI decision-making. Without transparency, comprehending the rationale behind AI decisions becomes challenging, eroding trust in these systems and potentially impeding their adoption.
Moreover, Explainable AI is increasingly vital in sectors like healthcare, finance, and transportation and HR where AI-driven decisions profoundly impact individuals’ lives. In such critical domains, understanding the rationale behind AI decisions is paramount.
What are the challenges of Explainable AI?
Achieving a balance between transparency and accuracy poses several hurdles in Explainable AI. In certain scenarios, enhancing transparency might entail compromising accuracy, as AI systems might need to relinquish some predictive capability to furnish explanations for their decisions. Hence, striking the appropriate equilibrium between transparency and accuracy is pivotal for the efficacy of Explainable AI.
Furthermore, the intricacy of AI systems compounds the challenge. Many AI algorithms exhibit considerable complexity, rendering them inscrutable to humans and impeding the development of lucid explanations. It’s even more true with recent model behing always more complicated and bigger than previous ones.
Lastly, the absence of standardized protocols adds another layer of complexity. Currently, there exists no consensus regarding the definition of Explainable” AI, making it arduous to assess the efficacy of various XAI methodologies and algorithms.
How is Explainable AI approached?
Researchers and policymakers are actively working to address the challenges of Explainable AI.
The European Union Artificial Intelligence Act (EU AIA), formally endorsed in December 2023, marks a significant legislative milestone in establishing a thorough framework for regulating AI systems across the EU. The Act underscores the EU’s dedication to striking a balance between promoting AI innovation and addressing the potential risks these technologies may pose to individuals and society.
Moreover, various Explainable AI (XAI) techniques and methodologies, such as interpretable machine learning, have been proposed by researchers. This approach concentrates on constructing models that are easily comprehensible for humans. Additionally, other techniques, like counterfactual explanations, offer insights into the decisions made by AI systems by demonstrating how altering input variables would impact the output.
XAI at Zortify: Bridging the Gap between AI and Human Understanding
At Zortify, we recognize the pivotal role of Explainable AI (XAI) in fostering an ethical and sustainable technology landscape. Our team is deeply motivated by the challenges and opportunities presented by the evolving field of XAI. Our design ethos revolves around prioritizing explainability, and we are dedicated to enhancing our products through our expertise, driving progress in the field as we move forward.
Conclusion
In conclusion, Explainable AI stands as a vital domain of research, aiming to mitigate the opacity inherent in AI algorithms and foster transparency and accountability in decision-making processes. The significance of Explainable AI cannot be overstated, and it is imperative that we tackle its associated challenges. Through enhanced transparency in AI systems, we can bolster trust and ensure that the decisions orchestrated by these systems uphold principles of ethics, fairness, and responsibility.
– Arrieta, A. B., et al. “Explainable Artificial Intelligence (XAI): Concepts, taxonomies, opportunities, and challenges toward responsible AI.” Information Fusion 58 (2020): 82-115.
– Lipton, Zachary C. “The mythos of model interpretability.” arXiv preprint arXiv:1606.03490* (2016).
– Wachter, S., Mittelstadt, B., & Russell, C. (2018). Counterfactual explanations without opening the black box: Automated decisions and the GDPR. Harvard Journal of Law & Technology, 31(2), 841-887.
– Doshi-Velez, F., & Kim, B. (2017). Towards a rigorous science of interpretable machine learning. arXiv preprint arXiv:1702.08608*.
– Gunning, D. (2017). Explainable artificial intelligence (XAI). Defense Advanced Research Projects Agency (DARPA).
*arXiv is a repository of scholarly articles in the fields of physics, mathematics, computer science, and other related disciplines. People commonly use the citation format “arXiv preprint arXiv:1702.08608” to reference articles that have been uploaded to arXiv before they are published in a peer-reviewed journal. In this format, “1702.08608” refers to the unique identifier or “e-print” number assigned to the article by arXiv.
Subscribe to our newsletter
Are you ready to shape the future of work and transform your HR strategies? – In our newsletter, we write about relevant topics between AI and HR and the fantastic new possibilities that artificial intelligence brings to modern People Management.